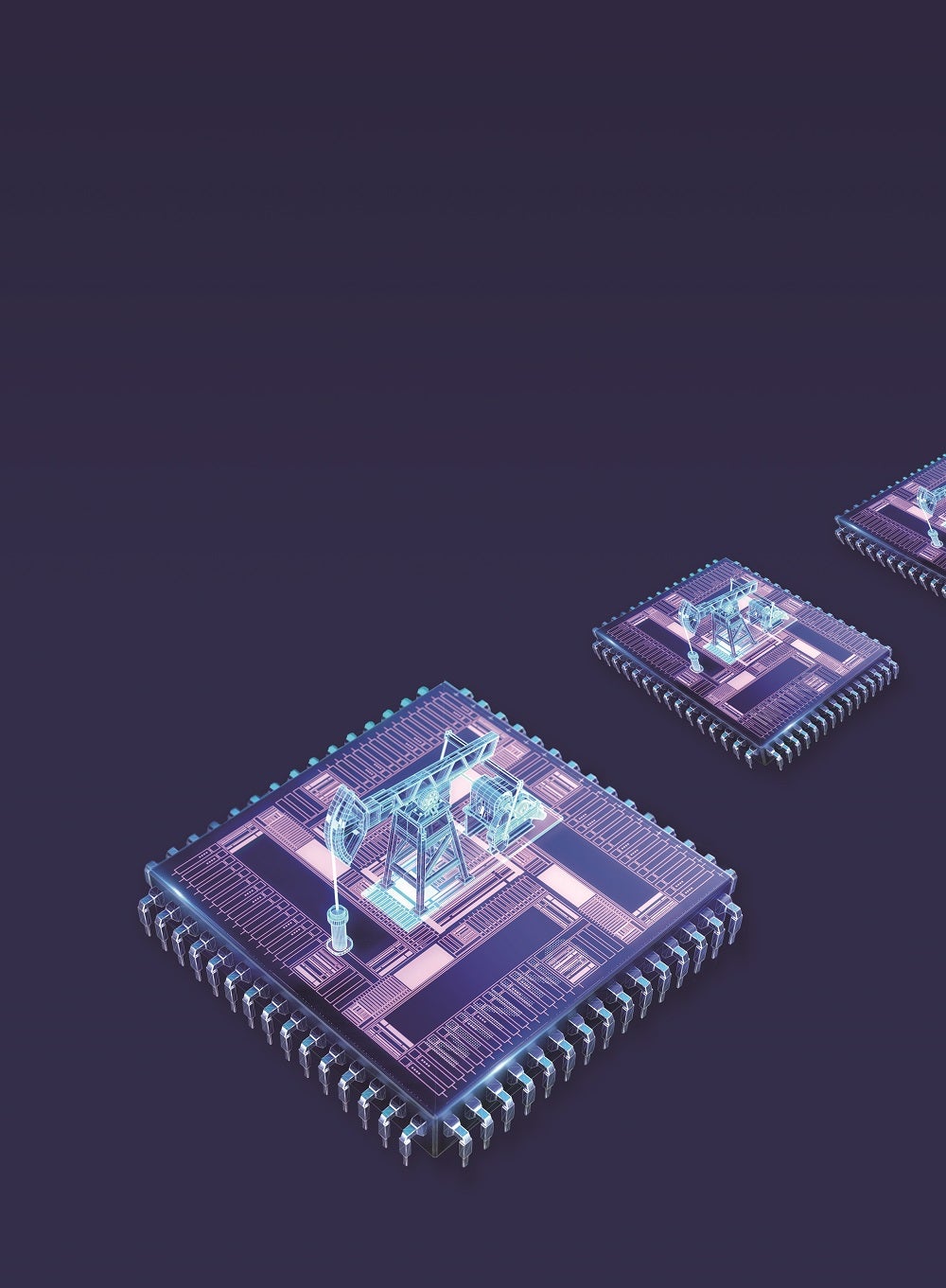
The bête noire of pipeline maintenance, corrosion costs the offshore oil and gas industry over $1 billion each year. AI-based predictive repairs, however, can help to dampen these costs, as Subrata Bhowmik, a senior subsea engineer at McDermott International, and Michael Smith, integrity analytics manager at ROSEN Group, tell Ross Davies.
According to estimates made by the National Association of Corrosion Engineers (NACE), pipeline corrosion costs the offshore oil and gas production industry roughly $1.4 billion a year.
Broken down, this amounts to $589 million in surface pipeline and facility expenses, $463 million in downhole tubing costs, with an additional $320 million in other capital expenditure pertaining to corrosion.
In its report, NACE, a not-for-profit group based in Texas, cited corrosion as “the primary factor affecting the longevity and reliability of gas and liquid transmission pipelines”. Put another way, pipeline corrosion is a scourge on the industry.
If not spotted early enough, corrosion can lead to pipeline fatiguing and, in worst-case scenarios, leaking – of which, the results can be devastating. This was demonstrated in August 2006 when BP was forced to shut down its operations in Prudhoe Bay, Alaska – at that time, the largest oilfield in the US – after it leaked 200,000gal of oil.
Heavy corrosion in a critical pipeline – which went undetected for five days – was discovered to be the dominant contributing factor to the spill by investigators. Its reputation tarnished, BP was later ordered to pay a record fine of $25 million. The incident served as a wake-up call to operators that they would be held accountable for the safety of their operations.
Thus, timely detection, followed by classification, remains the best means of prevention for pipeline operators all over the world.
Digital detection tacking the physical issues of identifying oil pipeline corrosion
The introduction of remotely operated underwater vehicles (ROVs) in recent years has undoubtedly improved corrosion inspection and management, although some explorers still rely on images taken manually by divers – a laborious process, particularly when concerning longer pipelines in deeper waters, where visibility is restricted.
Other methodologies currently on the market include electrical field mapping, field signature mapping and magnetic flux leakage. Non-destructive testing methods, such as eddy current testing (ECT), acoustic emission method (AEM) and ultrasonic testing (UT), have also become more popular of late.
However, the majority of these methods are subject to limitations, says Subrata Bhowmik, a senior subsea engineer at McDermott International. “The majority of current methods are intended for pipeline corrosion detection at, or near, the surface,” explains Bhowmik, who is based at the group’s London office.
“ECT is highly vulnerable to magnetic permeability in pipeline material, while AEM can only determine instantaneous assessment of damage, and cannot provide information on previously accrued damage. Therefore, it may not be suitable for corrosion rate prediction.
“Similarly, UT requires surface cleaning, and is highly dependent on operator experience with data processing.”
These technological shortcomings have prompted fresh research into automated image processing techniques as a means of affording explorers greater accuracy in corrosion detection, reducing maintenance costs into the bargain.
“The advancement of inspection and monitoring technologies for subsea pipelines brings the power of big data to the detection of pipeline corrosion,” Bhowmik continues. “Special processing methods play an important role in processing the significant amounts of data generated by the sensors installed for inspection.”
According to Bhowmik, the hope is that machine-learning algorithms could help to improve the quality of images taken in subsea inspections – in turn, leading to the more accurate prediction of pipeline corrosion.
- Pipeline maintenance is one of the biggest thorns in the midstream oil and gas sector (Credit: shinobi/Shutterstock.com)
Similarly, computer vision (CV), an AI-based image-processing technique, has the potential to assist operators in both corrosion identification and classification, based on the level of severity.
“This supports a continued focus on detailed information, and cost and time efficiencies by subsea inspection companies,” Bhowmik concludes.
The role of big data in protecting the pipeline environment is only set to grow, believes Michael Smith, integrity analytics manager at ROSEN Group, as companies strive to “squeeze the absolute last out of all the information that they’ve gathered”. Pipelines are already able to generate vast volumes of sundry data on everything from pressure and temperature to flow.
ROSEN supports a number of digital transformation initiatives within the pipeline industry, including the creation of an ‘integrity data warehouse’, effectively housing tens of thousands of pipeline data sets from all over the world under one roof. This, says Smith, would enable companies to create predictive models to support better decision-making. In other words, machine learning fed by big data.
“Traditionally, when you want to assess a pipeline, you collect data that pertains to that asset – and that asset alone,” he explains. “This means operators have not been able to look at different data all in one place, because it often takes a long time to do.
“But you can now get all that data into one place and then actually use it. This means you can look at all the assets together, and get additional information from other pipelines that might also be applicable to yours.”
Algorithms to determine if a subsea repair is required could lead to significant savings
McDermott, a global energy services company, is currently working on a new, computer vision-based pipeline inspection method. Even so, says Bhowmik, “further developments are required”, as the technology is “mainly intended for external corrosion detection, and has a limitation for a corrosion under insulation [CUI] case”.
Are there any other AI technologies and solutions that have caught his eye?
“Other technologies in development include fatigue or corrosion prediction using machine-learning algorithms based on historical inspection data,” Bhowmik answers. “There’s also the predictive modelling of pipeline internal corrosion using artificial neural networks.”
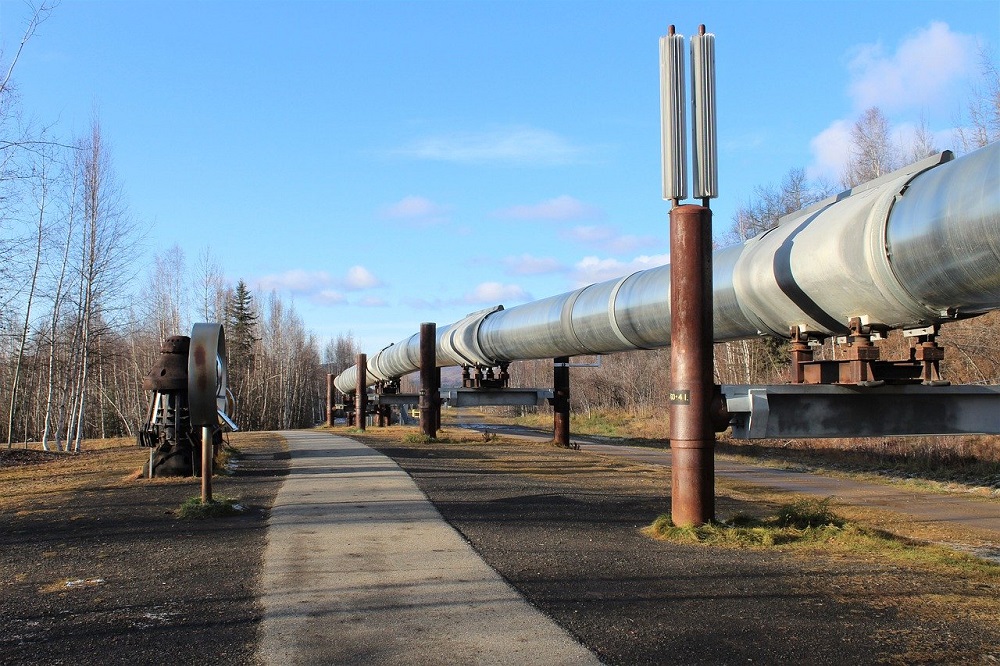
For oil and gas operators keen to lower their operating costs, the promise of making savings around pipeline maintenance is especially attractive. According to Smith, the application of machine-learning algorithms to determine whether or not a subsea repair is required could lead to significant savings.
“Focusing on the upstream sector alone, the costs associated with intervening with a subsea pipeline can be really quite extreme,” he says. “This means you need to be super accurate, prioritising the right kind of assessments. That’s what makes machine learning such an important technique.”
Bhowmik also believes AI-predictive repairs will be crucial in the future of pipeline optimisation. He refers to the rise of the ‘digital twin’ model – the concept whereby operators are able to access a virtual image of a pipeline at any point throughout its life cycle. In effect, the strategy provides a single platform for data, analytics, insight and diagnostics.
“The digital twin model for pipeline fatigue and corrosion monitoring is the future of pipeline inspection strategy,” he says. “Instead of a risk-based inspection strategy, predictive maintenance strategy can be deployed by implementing machine learning-based predictive analytics, real-time pipeline monitoring and cloud computing in a pipeline’s digital twin.
“This will save a significant amount of maintenance cost by avoiding unnecessary inspection. It will also design realistic inspection schedules, eventually helping oil and gas operators to reduce their operational costs.”
When, realistically, will this technology come into wider play? Smith believes AI-based predictive pipeline maintenance could become commonplace within the next decade, in line with ongoing digital disruption within the upstream oil and gas sector.
“With more and more digital technologies already coming into play, predictive maintenance is certainly going to be important in pipelines,” he says.
“It’s a long-term investment for companies and should become the norm in the next five to 10 years. I suspect we’ll begin with traditional machine learning before moving towards real-time predictive analytics that use deep learning.”
Like in any industry, some operators will be cagier than others in making such investments – returns might not necessarily be immediate. But those in charge of ageing assets – 45% of US crude oil pipelines are more than 50 years old, according to The American Petroleum Institute – scrimp on pipeline integrity at their peril. They must embrace the digital oilfield.
This article originally appeared in Issue 1, 2020, of World Expro. The full issue can be viewed here.