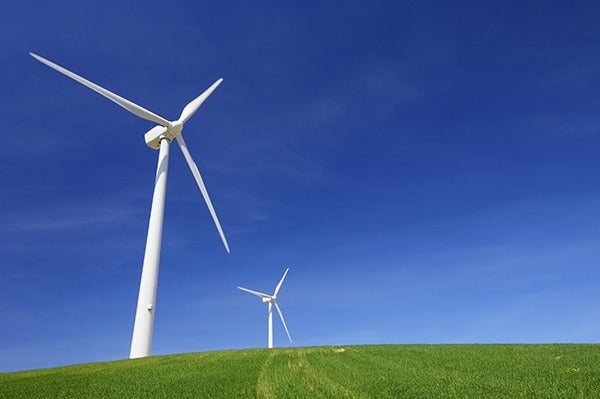
Clir Renewables said that a wind turbine would never generate its expected output one-hundred percent of the time, and its performance almost changes over time owing to various reasons. Though some of them are known but cannot be controlled or managed, such as fluctuating inflow conditions.
The software firm claims that they have created an underperformance detector for its software solution, by using layered machine learning, built on an advanced data model.
The detector is incorporated to the existing software and works along with other algorithms in the software to analyze the data and classify them based on the reason for the underperformance.
The company explained that the detector creates a synthetic event when turbine power output is below the historical mean for the specific wind speed.
This important information from this tool helps identify ongoing issues at a turbine, which are not indicated by the SCADA data, inflow conditions under which the turbine does not perform well, and the duration and lost energy associated with the underperformance.
In addition, the detector is also capable of highlighting a hardware or software configuration change that reduces power performance, along with removing the noise from the data leaving a clean set of data.
The clean data from the detector can be used to find the unknown causes, and create corrective actions.
Clir Renewables data scientist Selena Farris said: “With noise filled datasets the uncertainty of any conclusions that can be drawn on causes of underperformance will increase significantly, and in a lot of cases, issues can be completely masked by the noise.
“Utilizing the advances in machine learning, a well-structured data model, and deep domain expertise Clir software provides a tool to reduce this uncertainty, generating actionable insights for owners to increase performance and protect their assets from faults and failures.”